牵引变电站人体入侵检测的研究开题报告
2020-04-28 20:28:15
1. 研究目的与意义(文献综述)
1、目的及意义(含国内外的研究现状分析)
1.1研究背景及意义
随着我国轨道交通特别是高速客运专线和城市轨道交通的快速发展,牵引供电系统发展迅速。强大可靠的供电是保证轨道交通正常运行的基本要求,牵引变电站是牵引供电系统中的重要环节,其安全运行是系统可靠运行的前提。
2. 研究的基本内容与方案
毕业设计希望针对牵引变电站,在前人研究行人检测和智能电站的基础上,对人体入侵这一具体问题展开研究。
因此,考虑将研究分为三个阶段来进行:采集数据、行人检测模型的实现、设计特征或指标区分正常的工作人员和闯入人员。
根据以往学者的研究与工作,行人检测的数据集一般采用加州理工大学的行人数据集(caltech predestrain)法国自动化所的数据集(inria).关于牵引变电站的人体数据的搜集,一方面来自于网络图片,其次来自于生产实习时的所拍摄的照片。
3. 研究计划与安排
第一周 | 查阅相关文献资料,明确研究内容 |
第二周 | 了解设计题目的各种要求,分析设计要点、难点 |
第三周 | 了解行人检测国内外的发展状况和研究现状,熟悉其基本的检测原理,完成开题报告 |
第四周 | 针对不同的功能模块查阅资料,熟悉系统各部分的检测原理,初步确定系统设计方案 |
第五周 | 搜集行人数据,并对自己采集的数据进行标注 |
第六周 | 完成行人检测中的特征提取部分 |
第七周 | 完成行人检测中的变形处理部分 |
第八周 | 完成行人检测中的遮挡处理部分 |
第九周 | 完成行人检测中的分类部分 |
第十周 | 完成各个部分算法的比较,选出适用于本次毕设的算法组合 |
第十一周 | 设计正常人员与闯入人员的区分特征,并对产生的算法进行有效性验证 |
第十二周 | 撰写毕业设计论文初稿,并交导师审阅 |
第十三、十四周 | 完成论文的最终定稿,交指导教师和评阅教师评阅,并准备答辩 |
第十五周 | 进行毕业设计答辩 |
4. 参考文献(12篇以上)
[1] DALAL N, TRIGGS B. Histograms of Oriented Gradients for Human Detection; proceedings of the Computer Vision and Pattern Recognition, 2005 CVPR 2005 IEEE Computer Society Conference on, F, 2005 [C].
[2] VEDALDI A, GULSHAN V, VARMA M, et al. Multiple kernels for object detection; proceedings of the Proc IEEE International Conference on Computer Vision, F, 2009 [C].
[3] WANG X. An HOG-LBP human detector with partial occlusion handling [J]. Procieee Intconfon Computer Vision Kyoto Japan Sept, 2009, 30(2): 32-9.
[4] VIOLA P, JONES M J. Snow D.: Detecting pedestrians using patterns of motion and appearance, F, 2003 [C].
[5] DOLLáR P, TU Z, PERONA P, et al. Integral channel features [J]. 2009,
[6] GAO W, AI H, LAO S. Adaptive contour features in oriented granular space for human detection and segmentation; proceedings of the Computer Vision and Pattern Recognition, 2009 CVPR 2009 IEEE Conference on, F, 2009 [C]. IEEE.
[7] WALK S, MAJER N, SCHINDLER K, et al. New features and insights for pedestrian detection; proceedings of the IEEE Conference on Computer Vision and Pattern Recognition, F, 2010 [C].
[8] FELZENSZWALB P, MCALLESTER D, RAMANAN D. A discriminatively trained, multiscale, deformable part model [J]. Cvpr, 2008, 8:(1-8.
[9] PARK D, RAMANAN D, FOWLKES C. Multiresolution Models for Object Detection; proceedings of the European Conference on Computer Vision, F, 2010 [C].
[10] YAN J, ZHANG X, LEI Z, et al. Robust Multi-resolution Pedestrian Detection in Traffic Scenes; proceedings of the Computer Vision and Pattern Recognition, F, 2013 [C].
[11] FELZENSZWALB P F, HUTTENLOCHER D P. Pictorial Structures for Object Recognition [M]. Kluwer Academic Publishers, 2005.
[12] DESAI C, RAMANAN D. Detecting Actions, Poses, and Objects with Relational Phraselets [J]. Bmc Veterinary Research, 2012, 18(1): 418.
[13] YANG Y, RAMANAN D. Articulated pose estimation with flexible mixtures-of-parts; proceedings of the Computer Vision and Pattern Recognition, F, 2011 [C].
[14] BOURDEV L, MALIK J. Poselets: Body part detectors trained using 3D human pose annotations; proceedings of the IEEE International Conference on Computer Vision, F, 2009 [C].
[15] ENZWEILER M, EIGENSTETTER A, SCHIELE B, et al. Multi-cue pedestrian classification with partial occlusion handling; proceedings of the Computer Vision and Pattern Recognition, F, 2010 [C].
[16] WU T, ZHU S C. A Numerical Study of the Bottom-Up and Top-Down Inference Processes in And-Or Graphs [J]. International Journal of Computer Vision, 2011, 93(2): 226-52.
[17] SHET V D, NEUMANN J, RAMESH V, et al. Bilattice-based Logical Reasoning for Human Detection; proceedings of the Computer Vision and Pattern Recognition, 2007 CVPR '07 IEEE Conference on, F, 2007 [C].
[18] DOLLáR P, APPEL R, KIENZLE W. Crosstalk Cascades for Frame-Rate Pedestrian Detection; proceedings of the European Conference on Computer Vision, F, 2013 [C].
[19] HOSANG J, OMRAN M, BENENSON R, et al. Taking a Deeper Look at Pedestrians [J]. 2015, 4073-82.
[20] TIAN Y, LUO P, WANG X, et al. Pedestrian detection aided by deep learning semantic tasks; proceedings of the Proceedings of the IEEE Conference on Computer Vision and Pattern Recognition, F, 2015 [C].
[21] SERMANET P, KAVUKCUOGLU K, CHINTALA S, et al. Pedestrian Detection with Unsupervised Multi-stage Feature Learning; proceedings of the Computer Vision and Pattern Recognition, F, 2013 [C].
[22] OUYANG W, WANG X. A discriminative deep model for pedestrian detection with occlusion handling; proceedings of the Computer Vision and Pattern Recognition (CVPR), 2012 IEEE Conference on, F, 2012 [C]. IEEE.
[23] OUYANG W, WANG X. Joint Deep Learning for Pedestrian Detection; proceedings of the IEEE International Conference on Computer Vision, F, 2013 [C].
[24] 王旭. 无人值守变电站智能视频监测系统的设计与实现 [D]; 华北电力大学, 2014.
[25] 张朝龙, 王硕, 梁凯, et al. 人眼视觉识别技术在变电站视频监控的应用 [J]. 电气应用, 2013, s1): 496-7.
[26] 武爱敏. 变电站视频中工作人员的识别 [D]; 华北电力大学(北京), 2017.
最新文档
- 华北地区夏季降水多年、少年近地层水汽场及风场分布特征开题报告
- 天山地区典型流域降雪分布研究文献综述
- 2016年台风狮子山路径突变原因的初步分析开题报告
- 北京和寿县地区边界层急流观测与模型的对比文献综述
- 具有双Kelvin波结构MJO活动的再分析开题报告
- 基于单片机的温控风扇设计文献综述
- 1961-2015年江苏省气候资源的时空分布规律开题报告
- 智能搬运机器人文献综述
- 基于类型的电影推荐系统文献综述
- Bi6S2O15的合成及其降解有机污染的研究开题报告
- 不确定时滞奇异摄动系统的鲁棒控制器设计文献综述
- 小型双模SIW滤波器的研究与设计开题报告
- 我国绿色出行政策执行研究——以南京市为例文献综述
- 试论李碧华小说中的爱情悲剧开题报告
- 基于FPGA的电子相册设计文献综述
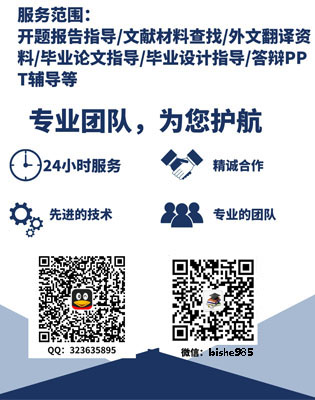