基于深度学习的论文摘要写作辅助模型开题报告
2020-04-12 16:03:20
1. 研究目的与意义(文献综述)
2005年,scigen[1]横空出世,开创了人工智能写论文、文章的先河。
虽然scigen实现的技术以现在的观点来看十分简略,而且发明它的三位mit的研究生本身也只是以恶搞为目的,但用人工智能来辅助人类进行写作这个思想则从此进入实际可行的范围。
从此,学者们开始对人工智能自动辅助写作展开了美好的篇章。
2. 研究的基本内容与方案
需要实现的功能:
1 抓取论文数据
2 根据论文数据的上下文训练lstm神经网络及编码器-译码器模型
3 获取一句用户输入为原始句子
4 将这个句子导入训练好的编码器-译码器模型,得到对应当前句子的句子
5 重复第4步,直到获取到足够的句子构成一段话。
2.2技术方案:
本文拟采用以下几个技术方案实现目标。
首先,为了提高准确度,即解决问题(1),应采用深度学习及其相关方法作为基本框架。
3. 研究计划与安排
(1)2018/1/14—2018/2/22:确定选题,查阅文献,外文翻译和撰写开题报告;
(2)2018/2/23—2018/4/30:系统架构、程序设计与开发、系统测试与完善;
(3)2018/5/1—2018/5/25:撰写及修改毕业论文;
(4)2018/5/26—2018/6/6:准备答辩。
4. 参考文献(12篇以上)
1. Stribling, J., M. Krohn, and D. Aguayo, Scigen-an automatic cs paper generator. 2005.
2. Parveen, D. and M. Strube. Integrating Importance, Non-Redundancy and Coherence in Graph-Based Extractive Summarization. in IJCAI. 2015.
3. Erkan, G. and D.R. Radev, Lexrank: Graph-based lexical centrality as salience in text summarization. Journal of Artificial Intelligence Research, 2004. 22: p. 457-479.
4. Liu, W., et al., Semantic summary automatic generation in news event. Concurrency and Computation: Practice and Experience, 2017. 29(24).
5. Zhang, C., et al. Text summarization based on sentence selection with semantic representation. in Tools with Artificial Intelligence (ICTAI), 2014 IEEE 26th International Conference on. 2014. IEEE.
6. Chen, J. and H. Zhuge, Automatic generation of related work through summarizing citations. Concurrency and Computation: Practice and Experience, 2017.
7. Wanner, L., S. Verlinde, and M. Alonso Ramos. Writing assistants and automatic lexical error correction: word combinatorics. in Electronic lexicography in the 21st century: thinking outside the paper. 2013. Trojina, Institute for Applied Slovene Studies/Eesti Keele Instituut.
8. Crossley, S., Z. Cai, and D.S. McNamara. Syntagmatic, paradigmatic, and automatic n-gram approaches to assessing essay quality. in Twenty-Fifth International FLAIRS Conference. 2012.
9. Liu, M., R.A. Calvo, and V. Rus, G-Asks: An intelligent automatic question generation system for academic writing support. Dialogue amp; Discourse, 2012. 3(2): p. 101-124.
10. Kannan, A., et al. Smart reply: Automated response suggestion for email. in Proceedings of the 22nd ACM SIGKDD International Conference on Knowledge Discovery and Data Mining. 2016. ACM.
11. Ma, X. and E. Hovy, End-to-end sequence labeling via bi-directional lstm-cnns-crf. arXiv preprint arXiv:1603.01354, 2016.
12. Sutskever, I., O. Vinyals, and Q.V. Le. Sequence to sequence learning with neural networks. in Advances in neural information processing systems. 2014.
13. Cho, K., et al., Learning phrase representations using RNN encoder-decoder for statistical machine translation. arXiv preprint arXiv:1406.1078, 2014.
14. Wang, S. and J. Jiang, Machine comprehension using match-lstm and answer pointer. arXiv preprint arXiv:1608.07905, 2016.
15. Yang, Z., et al. Hierarchical attention networks for document classification. in Proceedings of the 2016 Conference of the North American Chapter of the Association for Computational Linguistics: Human Language Technologies. 2016.
16. Andor, D., et al., Globally normalized transition-based neural networks. arXiv preprint arXiv:1603.06042, 2016.
最新文档
- 华北地区夏季降水多年、少年近地层水汽场及风场分布特征开题报告
- 天山地区典型流域降雪分布研究文献综述
- 2016年台风狮子山路径突变原因的初步分析开题报告
- 北京和寿县地区边界层急流观测与模型的对比文献综述
- 具有双Kelvin波结构MJO活动的再分析开题报告
- 基于单片机的温控风扇设计文献综述
- 1961-2015年江苏省气候资源的时空分布规律开题报告
- 智能搬运机器人文献综述
- 基于类型的电影推荐系统文献综述
- Bi6S2O15的合成及其降解有机污染的研究开题报告
- 不确定时滞奇异摄动系统的鲁棒控制器设计文献综述
- 小型双模SIW滤波器的研究与设计开题报告
- 我国绿色出行政策执行研究——以南京市为例文献综述
- 试论李碧华小说中的爱情悲剧开题报告
- 基于FPGA的电子相册设计文献综述
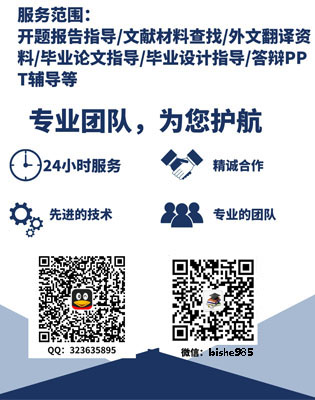